Bio
I’m CTO of EfficientAI, a stealth-stage startup that is designing ultra-low-power microcontrollers using technology I developed during my PhD. I recieved my PhD (2017-2022) in Computer Science from Carnegie Mellon, where I was advised by Brandon Lucia and Nathan Beckmann. My dissertation was titled “Programmable, Energy-minimal Computer Architectures.” Prior to CMU, I completed my bachelors in computer science at Columbia University. Outside of my academic interests, I enjoy riding my bike, walking & running outdoors, audiobooks & podcasts, cooking, Formula 1 and last, but certainly not least, rooting for Arsenal!
Research
My PhD research primarily focused on improving the energy-efficiency of low- and ultra-low-power sensor devices. These devices enable many new applications, including in- and on- body medical implants, wildlife monitoring, tiny, chip-scale satellites, and civil infrastructure monitoring. Energy-efficiency is the key determinant for how deployed devices in these applications will perform: for devices powered by batteries, energy efficiency determines the lifetime of the device and for devices sourcing energy harvested from the environment, energy efficiency determines which applications are feasible and the duty cycle (how long it takes to recharge) of the device.
Commercially-available off-the-shelf systems in this domain are not energy-efficient and are severely resource constrained, often possessing mere kilobytes of main memory. Unfortunately, offloading computation from a sensor device to a more powerful edge device or to the cloud is infeasible or impractical because communication is vastly more expensive than computation. These problems lead to two directions of research: first, designing intelligent applications that can make decisions on the devices, but do not require significant resources, and second, designing new, more energy-efficient computer architectures to better support these new classes of applications.
Please refer to dissertation, my publications as well as intermittent.systems and cmu-corgi for more information.
RipTide: A Programmable, Energy-minimal Dataflow Compiler and Architecture
CGRAs are an ideal choice to improve the energy efficiency of ULP sensor systems while maintaining a high-degree of programmability. Unfortunately, nearly all prior CGRAs support only computations with simple control flow, causing an Amdahl efficiency bottleneck as non-trivial fractions of programs must run on an inefficient von Neumann core.
Riptide addresses this bottleneck; it is a co-designed compiler and ULP CGRA architecture that achieves both high programmability and extreme energy efficiency. RipTide provides a rich set of program primitives that support arbitrary control flow and irregular memory accesses. It also enforces memory ordering through careful analysis at compile-time, eliminating the need for expensive tag-token matching on fabric. RipTide also observes that control-flow operations are simple, but prevalent, so allocating them valuable PE resources is wasteful. Instead, RipTide offloads these operations into its on-chip network, reusing existing switch hardware. RipTide compiles applications entirely written in C while saving 25% energy v. SNAFU.
SNAFU: An ULP, Energy-Minimal CGRA-generation Framework and Architecture
SNAFU is a framework for generating ultra-low-power, energy-minimal course-grain reconfigurable arrays. It is designed from the ground-up to maximize flexibility while minimizing energy. For flexibility, SNAFU provides a standardized interface for processing elements, allowing for the easy integration of custom logic across the stack. For minimizing energy, SNAFU eliminates the primary source of energy-inefficiency in the prior state-of-the-art design, MANIC. Rather than share pipeline resources, SNAFU implements spatial-vector-dataflow execution, configuring a processing element once for the entirety of a kernel’s execution. This minimizes transistor toggling from control and data signals.
MANIC: A Vector-Dataflow Architecture for Ultra-low-power Embedded Systems
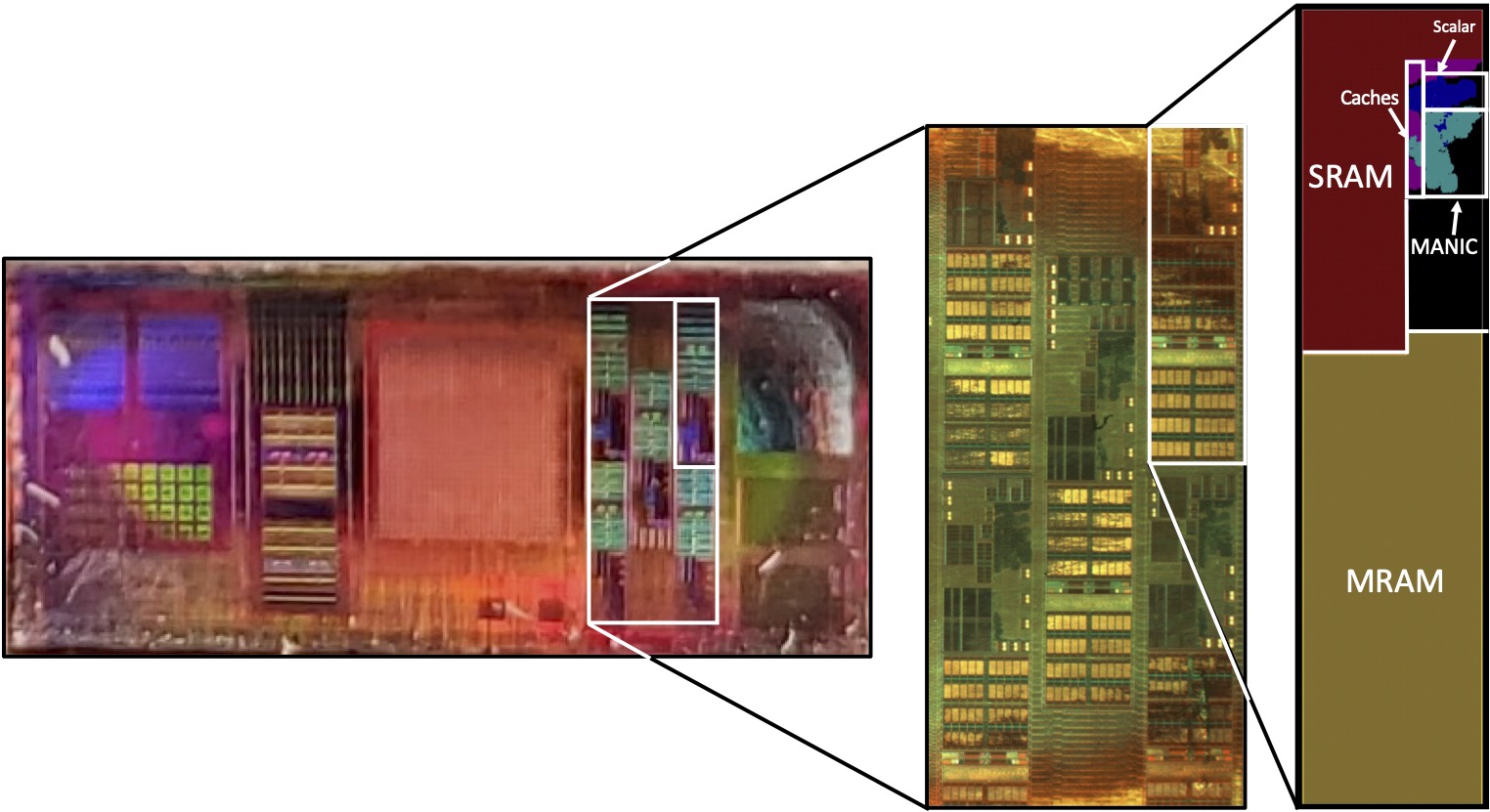
Commercially-available off-the-shelf microcontrollers are energy- inefficient. Instruction supply energy and data supply energy (RF accesses) are two primary sources of energy-inefficiency. Vector execution is one way to improve energy-efficiency by amortizing instruction fetch and decode. However, traditional vector architectures require a vector register file with expensive access energy. We introduce MANIC, a loosely-coupled vector co-processor that implements a new execution model called vector-dataflow execution. Vector-dataflow execution eliminates the majority of vector register file accesses by identifying opportunities for dataflow and forwarding values from producers to consumers. In typical vector execution, control completes an entire instruction’s worth of computation before moving onto the subsequent instruction. MANIC flips this around. MANIC considers a collection of instructions at once, exposing opportunities for dataflow. In vector-dataflow execution, control transfers executes the first elements of the instructions, then the second elements, then the third and so on.
In 2021, we fabricated MANIC in Intel 22FFL. The chip draws just 19 μW and achieves a maximum efficiency of 256MOPS/mW.
Inference on Intermittent Embedded Systems (GENESIS, SONIC, TAILS)
Continuous streaming of raw sensor data from a node to a central hub is inpractical for an intermittent device because communication is expensive and oftentimes infeasible. Thus, it is important to make the most out of any opportunity to communicate. Machine learning allows us to effectively determine whether sensor data is relevant and should or should not be transmitted. SONIC and TAILS are two runtime systems that make inference on intermittent devices correct and efficient. SONIC is entirely software-based, while TAILS relies upon hardware acceleration available on a variety of new MCUs.
Fellowships/Awards
Apple Scholar in AI/ML
Prestigious 2-year fellowship awarded by Apple to twelve PhD students globally. Fellowship was awarded in recognition of work on on-device machine-learning. Please see ml@apple for more information.
Publications
- RipTide: A Programmable, Energy-minimal Dataflow Compiler and Architecture
Graham Gobieski, Souradip Ghosh, Marijn Heule, Todd Mowry, Tony Nowatzki, Nathan Beckmann, Brandon Lucia,
MICRO 2022 [paper] - SNAFU: An Ultra-Low-Power, Energy-Minimal CGRA-Generation Framework and Architecture
Graham Gobieski, Oguz Atli, Ken Mai, Brandon Lucia, Nathan Beckmann
ISCA 2021 [paper] - MANIC: A Vector-Dataflow Architecture for Ultra-Low-Power Embedded Systems
Graham Gobieski, Amolak Nagi, Nathan Serafin, Mehmet Meric Isgenc, Nathan Beckmann, Brandon Lucia
MICRO 2019 [paper] - Intelligence Beyond the Edge: Inference on Intermittent Embedded Systems
Graham Gobieski, Brandon Lucia, and Nathan Beckmann
ASPLOS 2019 [paper] [ArXiv] - Intermittent Deep Neural Network Inference
Graham Gobieski, Nathan Beckmann, and Brandon Lucia
SysML (now MLSys) 2018 [paper] - Shuffler: Fast and Deployable Continuous Code Re-Randomization
David Williams-King, Graham Gobieski, Kent Williams-King, James P Blake, Xinhao Yuan, Patrick Colp, Michelle Zheng, Vasileios P Kemerlis, Junfeng Yang, William Aiello
OSDI 2016 [paper] - Clickable poly (ionic liquids): A materials platform for transfection
Jessica Freyer, Spencer Brucks, Graham Gobieski, Sebastian Russell, Carrie Yozwiak, Mengzhen Sun, Zhixing Chen, Yivan Jiang, Jeffrey Bandar, Brent Stockwell, Tristan Lambert, Luis Campos
Angewandte Chemie 2016 [paper]